Structured Data
Structured Data refers to any data that resides in a fixed field within a record or file. This includes data contained in a relational databases or spreadsheets. Structured data is a general name for all markups that abides by a predetermined set of rules. These rules include defining types of data and also the relationships between them. Structured Query Language (SQL) is most commonly used to manage structured data. SQL helps us perform several operations to analyze the data and fetch desired results. These operations include search, insert, update, delete and others.
Unstructured Data
Unstructured Data refers to any data that does not resides in traditional row-column database. Unstructured data includes files such as text files or multimedia content (e.g. emails, videos, photos, web pages, etc). Unstructured data is not relational and does not fit into any pre-defined data models. There are multiple techniques such as data mining, data analytics, NLP to process the unstructured data and try to find patterns in the data.
Structured vs Unstructured Data
Structure Data
|
Unstructured Data
|
|
Representation
|
Discrete –
rows and columns
|
Less defined boundaries and less easily addressable
|
Storage
|
DBMS or file formats
|
Unmanaged file structures
|
Metadata
|
Syntax
|
Semantics
|
Integration Tools
|
ETL
|
Batch processing or manual data entry
|
Standards
|
SQL, ADO.net, ODBC
|
XML, SMTP, SMS
|
Examples
|
Sales Data, Sensor Data
|
Images, Videos, Natural language text
|
Data Types and Data Volume
Currently all organizations are concerned with Big Data. Big data refers to extremely large datasets that are difficult to analyze with traditional tools. Big data can include both structured and unstructured data, but IDC estimates that 80 percent of big data is unstructured data. And the amount of Big Data is increasing continuously in organizations, more faster than the structured data.
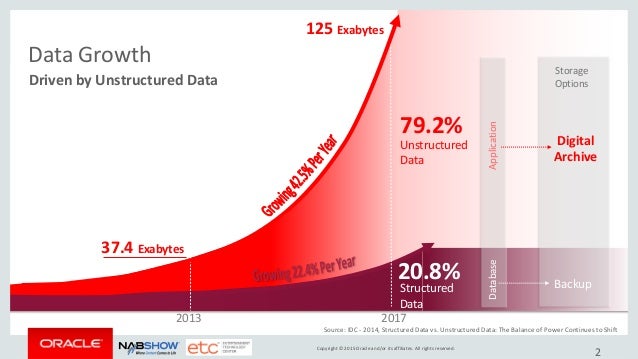
Since the volume of unstructured data and structured data is growing so rapidly, organizations are looking for technological solutions to store this data and manage the data. These solutions include both hardware and software solutions that enable enterprises to make efficient use of the available storage space. This is where data warehouse concept comes into play. A data warehouse is a database that is designed to maintain historical data and analyze the data to gain a better understanding of the business and improve. A data warehouse can be used to enable Business intelligence activities, helping users to understand and enhance organization's performance. A data warehouse environment can include an extraction, transportation, transformation, and loading (ETL) solution, statistical analysis, reporting, data mining capabilities, client analysis tools, and other applications that manage the process of gathering data, transforming it into useful, actionable information, and delivering it to business users.
Limitations of Data Warehouse in terms of Data Analysis
- Data load process that includes extraction, transformation and loading of the historical data might take longer time and hence, the time to develop the data warehouse will significantly increase.
- During data loading process inconsistent data might be loaded and result in performance degradation.
- In some cases, important information related to the business process under analysis is not captured by the source system but maybe important for strategic decision making.
- Integration of data from various disparate sources is a highly complex task. Also, a different tool performs each task within a data warehouse and integration of all these tools also increases the complexity of implementing a data warehouse.
- Data warehouses are high maintenance systems. If there are changes in the business processes (and hence, the data) then it will result in a change in the data warehouse and would result in very high maintenance costs.
Future of Data Warehouse
Data warehousing has never been more valuable before and is been used widely across enterprises. Making decisions based on data is so fundamental and obvious that the current generation of business users and data warehouse designers can’t imagine a world without access to data. Traditional data warehouse ETL has become too slow, too complicated, and too expensive to address the torrent of new data sources and new analytic approaches needed for decision making. The new ETL environment is already looking drastically different by supporting data feeds of huge bandwidths and multiple data sources.
Also enterprises moving to single unified system to access data which is achieved using Cloud-based technologies. Companies looking for cloud-based data warehouse might become the norm. The cloud based solutions provides flexibility, performance improvements and analytic tools support such as BI consulting, Data Analytics, and Big data, providing additional reason for cloud based data warehouse. Cloud based solution will also reduce maintenance and management costs.
No comments:
Post a Comment